How Conode Creates Dynamic Ontologies for Your Knowledge Graph
- shona060
- Jan 30
- 5 min read
Updated: Apr 15
Introduction
When building Knowledge Graphs, companies have historically spent months defining the schemas only to find them outdated by the time they’re deployed.
But what if you could skip the upfront work and get straight to exploration and insights?
In this blog, Conode CTO Nils Goldbeck traces the evolution of ontologies, from early computer vision to GenAI, and show how Conode’s dynamic, schema-on-read approach lets you build, refine, and extend ontologies—without the complexity.

Key Takeaways
With Conode, you can ingest data instantly, iterate effortlessly, and gain insights faster. 🚀
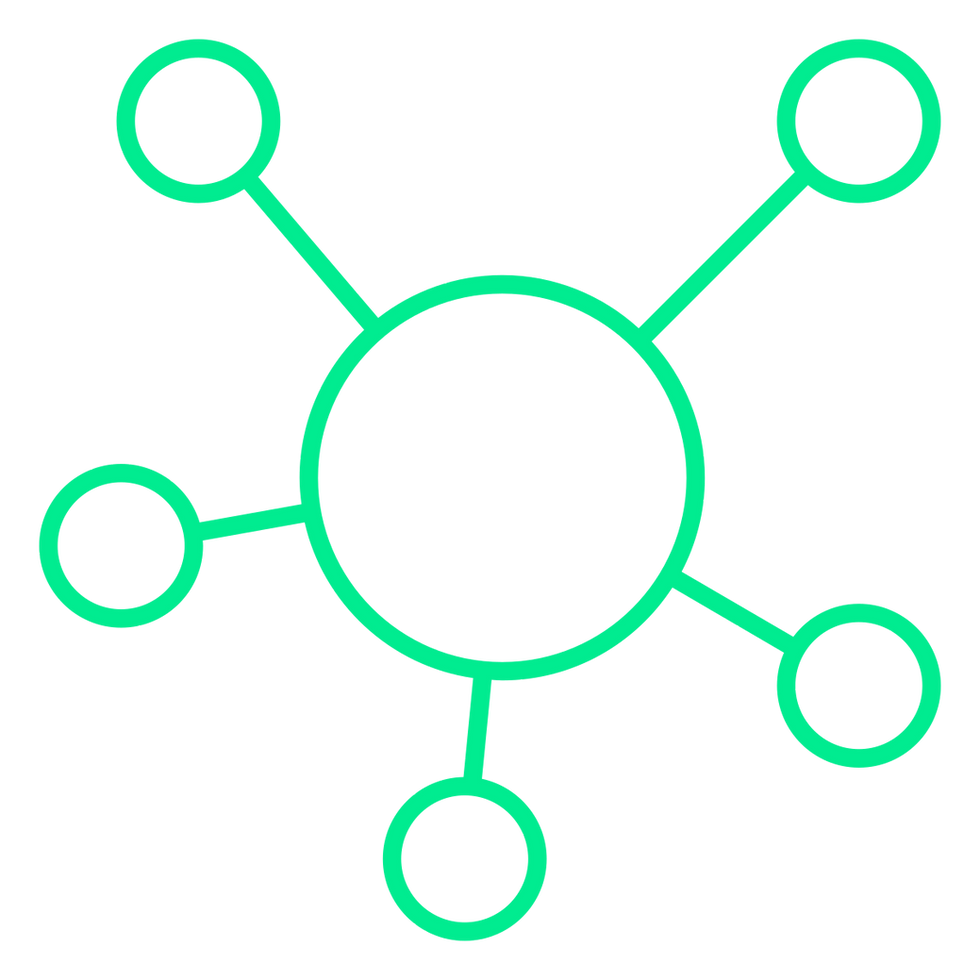
✅ No rigid schemas – The graph emerges from the data
✅ Automated ontology creation – Constructed on the fly
✅ Dynamic & adaptable – Refine structure as needs evolve
✅ No-code interaction – Modify via simple UI or natural language

What is an Ontology?
In philosophy, ontology means the "study of being" and describes the process of dividing reality into foundational building blocks, or categories that characterise the world in the most general terms.
Similarly, in information science the development of an ontology (aka ontology engineering) means the formal definition of categories, for example, categories of entities, concepts, relations or properties. Ontologies have been an important topic in the field of automated reasoning for many decades and some practitioners even refer to it simply as the "O-word" because of the central role of ontologies in knowledge-based systems.
The Evolution of Ontologies in Object Detection
During the deep learning revolution of the 2010s, many of us were amazed by the astonishing capabilities of computer vision models but not much attention was given to the underlying ontologies. These ontologies quickly turned out to be one of the limiting factors of computer vision applications, like autonomous driving.
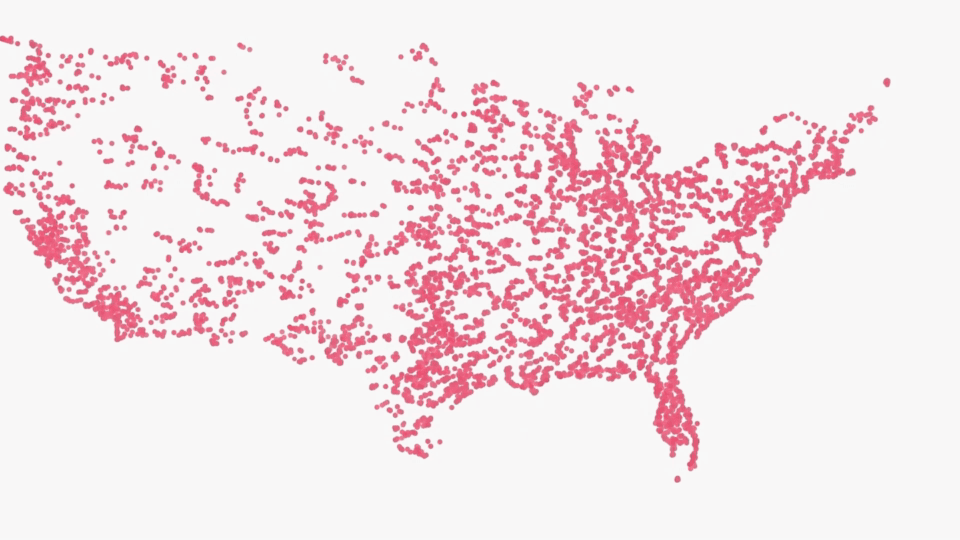
Object detection models can only recognise categories of objects that were included in the ontology of the training data. For example, the widely used COCO dataset contains 91 object categories, like car, bus, truck or bicycle. But there are many other types of vehicles that do not naturally fit into this ontology, for example, vans, golf carts or e-scooters. An object detection model that has been trained on COCO data cannot recognise these vehicle types unless it is re-trained, for which new training data needs to be gathered and labelled using the refined ontology. This illustrates a general difficulty with ontologies: They introduce a rigidity that makes the entire knowledge system very inflexible and costly to adapt when requirements evolve.
Ontologies in GenAI
In contrast, GenAI models do not at all rely on such schematic ontologies that need to be explicitly defined based on a priori knowledge.
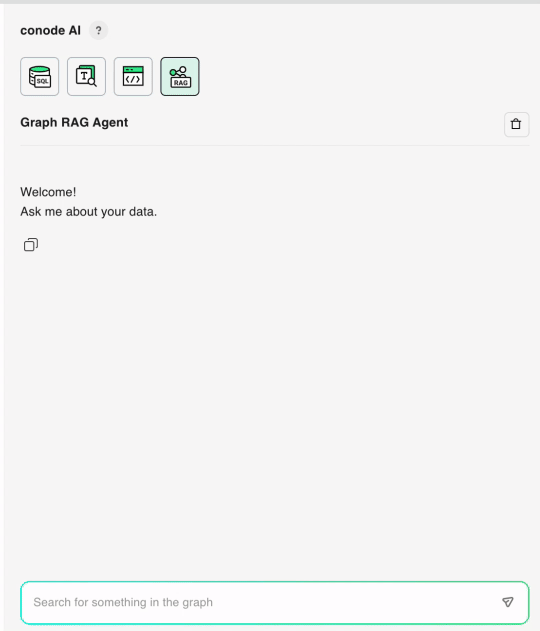
Yet, you can ask ChatGPT for a list of vehicle categories and it will give you a very comprehensive ontology, much more comprehensive than most SOTA object detection models. And text-to-image models like DALL-E have no problem creating images of any of these vehicle types, including golf carts or cement trucks.
Instead of relying on formal ontologies, GenAI models are able to use the most universal ontology that is also closest to human intelligence: natural language.
What does this mean for Knowledge Graphs?
Traditionally, Knowledge Graphs also relied on rigid schemas.
Before loading any data into the graph, Knowledge Graph engineers would have to define a graph schema, meaning a dictionary that defines the types of entities and relationships that nodes and edges represent. Often, the graph schema also defines certain attributes of nodes and edges, leading to a data model that is referred to as property graph. Other approaches for formalising ontologies for Knowledge Graphs include the Resource Description Framework Schema (RDF Schema) or the Web Ontology Language (OWL).
What do these conventional approaches of ontology engineering for Graphs have in common?
Formalised, rigid schemas
Require the input of subject-matter experts
Time Consuming
So much so that many companies consider the upfront cost of developing an ontology prohibitive. And for those who do endeavour on an ontology engineering project..
Despite investing extensive resources, companies often find that their ontology is already outdated by the time a Knowledge Graph finally gets deployed in production.
The Conode Approach
Conode works with dynamic ontologies.
The core schema for our Knowledge Graphs is very simple: a node can have two attributes (”label” and “content”), and an edge has a numeric weight attribute. Everything else is represented with the graph structure and therefore highly dynamic and adaptable.
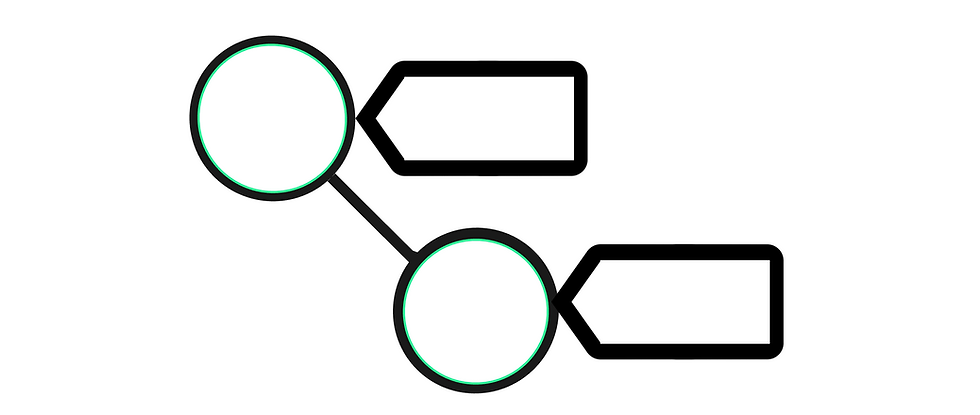
Manually building an ontology with existing tools is time consuming and inflexible. With Conode, you simply upload your data and let the graph skeleton emerge.
The Conode pure graph structure approach has a number of key advantages:
Low setup costs: We totally eliminated the lengthy process of upfront ontology engineering. Conode users start interacting with the Knowledge Graph right from the beginning and do not have to make difficult decisions about the scope and content of ontologies while not even having full visibility of that data yet.
Adaptability: It is very common that requirements for business intelligence or similar knowledge-heavy projects change as the analysts gain a better understanding of the domain, new data becomes available, or the project’s objectives shift due to external circumstances. Conode’s dynamic approach to ontologies makes it very easy to adjust the Knowledge Graph, e.g. by adding or fusing categories and creating multi-level annotation trees.
Scalability: As Knowledge Graphs grow, static ontologies often become a limiting factor and it is not uncommon that projects have to go back to square one and start with a completely new ontology when they reach a new level of scale. In Conode, the ontology is contained in the graph and can easily be updated on the fly as new data is ingested and processed.
Compatibility: A dynamic schema is ideally suited for fusing together data from many heterogenous sources that may all have their own ontologies. Conode’s patented methods for visualizing and manipulating graph databases are able to transform the schemas of relational databases and many other data formats (including CSV, JSON, PDF, Parquet, JPEG, PNG, MP4) into dynamic graph structure schemas (more details here).
How does a Dynamic Schema Approach Work in Practice?
Imagine you want to investigate the California Department of Motor Vehicles (DMV) autonomous vehicle collision reports. This data is mostly structured, containing information about the vehicle, weather conditions, injuries and damages.
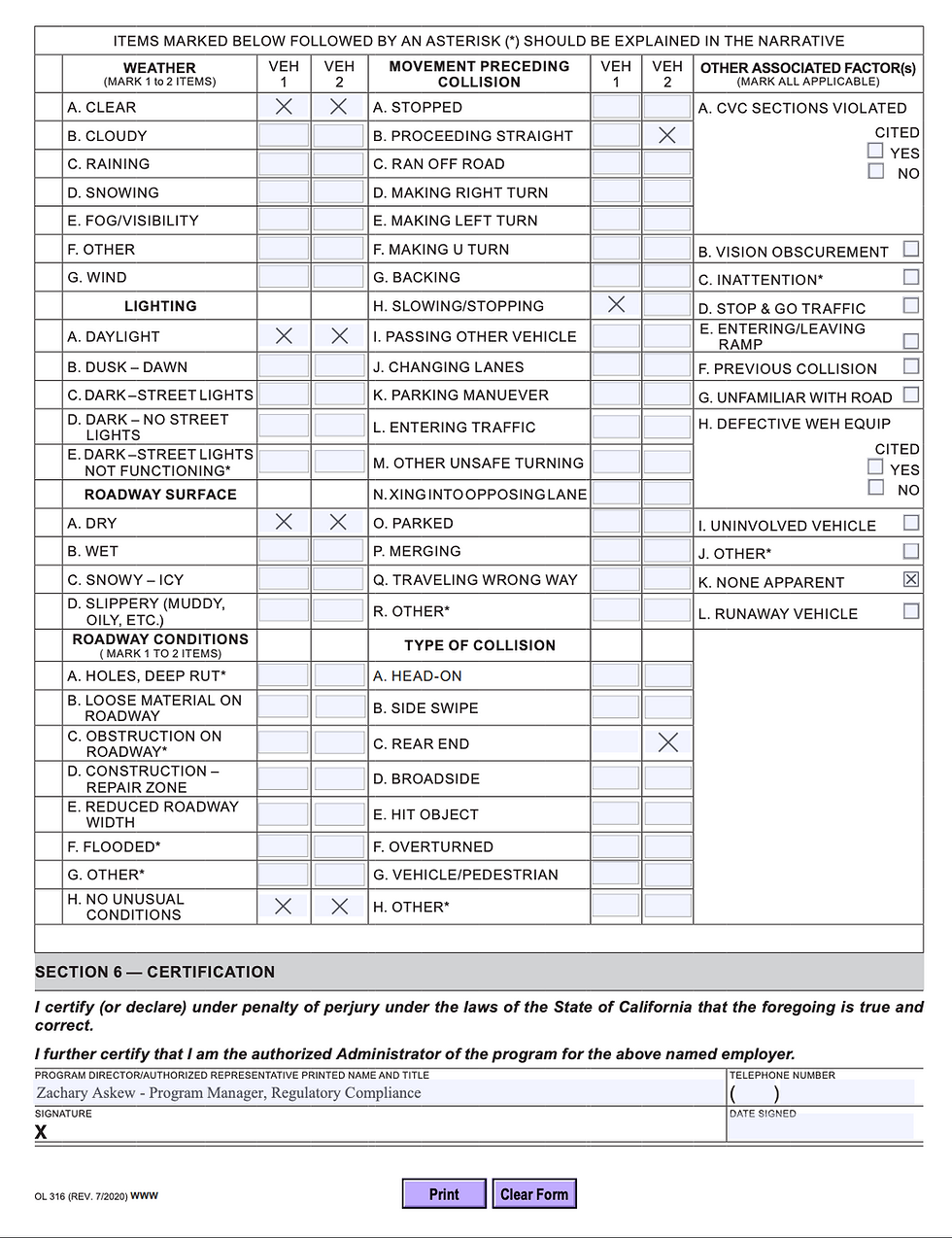

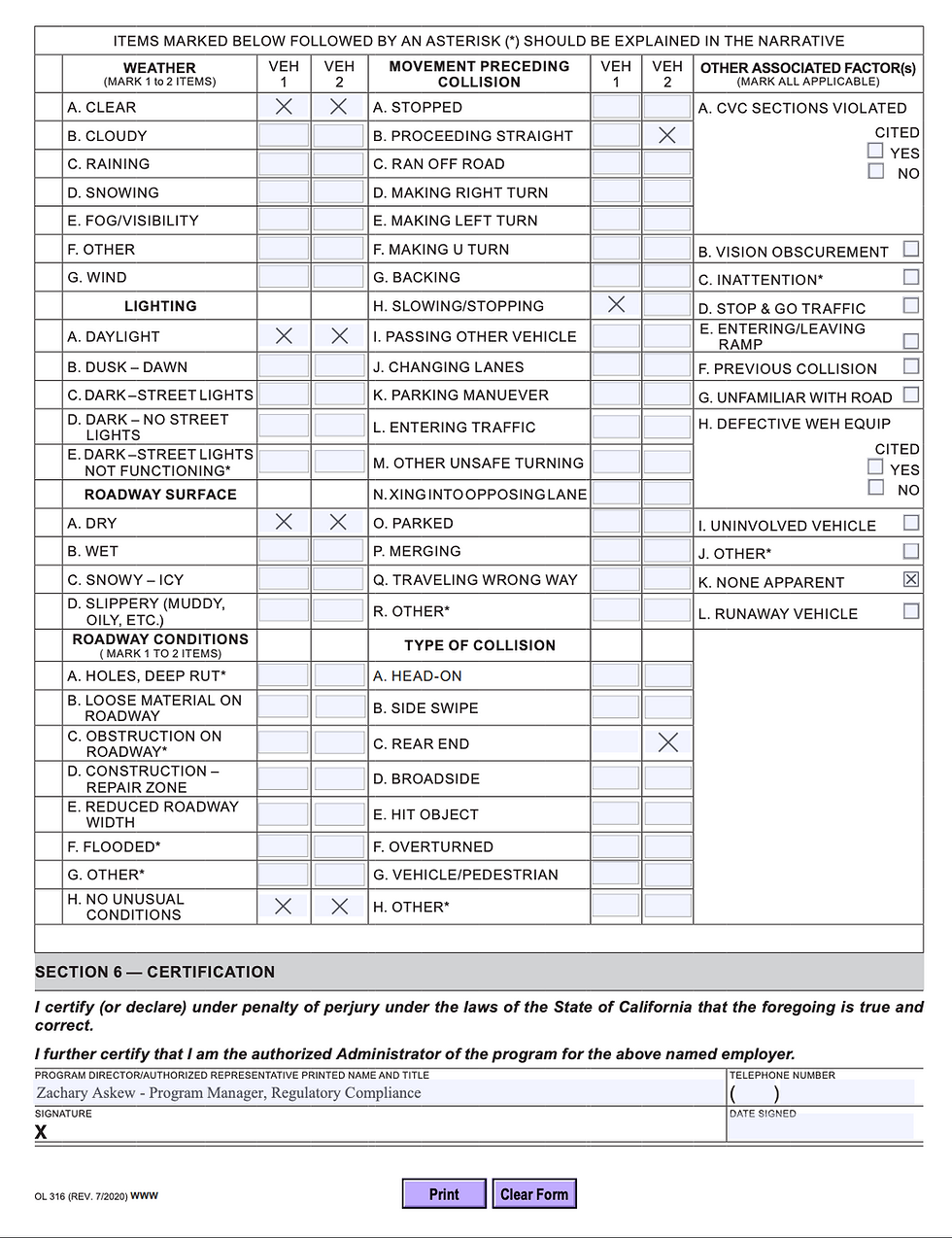
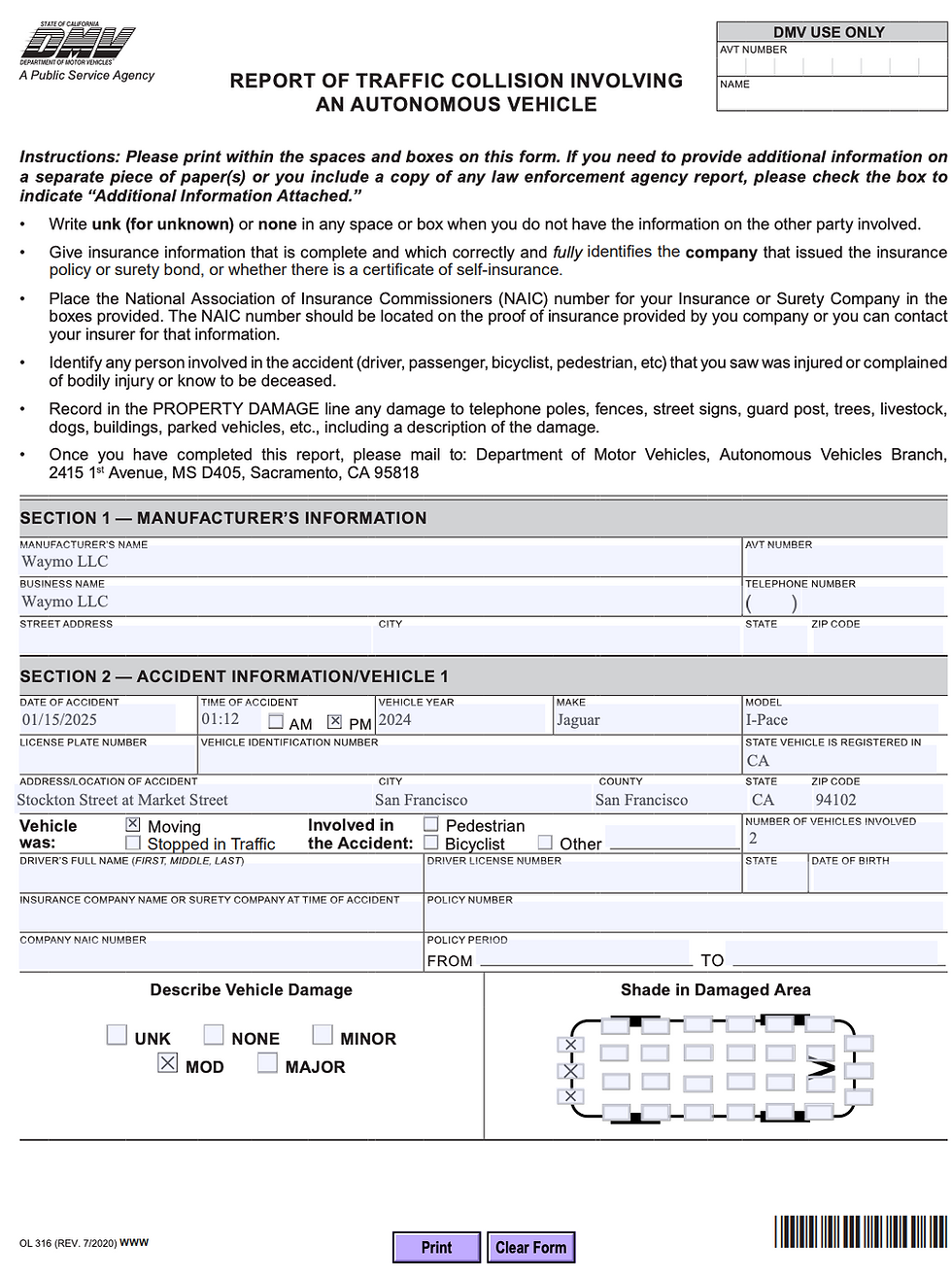
California Department of Motor Vehicles (DMV) autonomous vehicle collision reports
When the data is imported into Conde, the original ontology is automatically transformed into graph structure and you can start exploring the weather conditions or distribution of injuries right away.
You might then want to plot the accident locations on a map, but you realise that the data only contains location descriptions like “Florida St approaching Treat Av”. Conode’s geocoding agent can look up the latitude and longitude for these locations and extend the ontology directly in the graph so that it is then possible to create a spatial view of the collisions.
Imagine you further want to better understand the risk of road debris for AVs. Objects are not contained in the original ontology but the dataset contains accident descriptions in natural language that sometimes mention such obstacles. You can ask Conode’s Extract Agent to search for such information and it will suggest to add new feature to the graph, as shown below. In this case, the agent suggests to add a binary field for “Debris or Obstacle Description” and a categorical variable “Debris or Obstacle Description”.
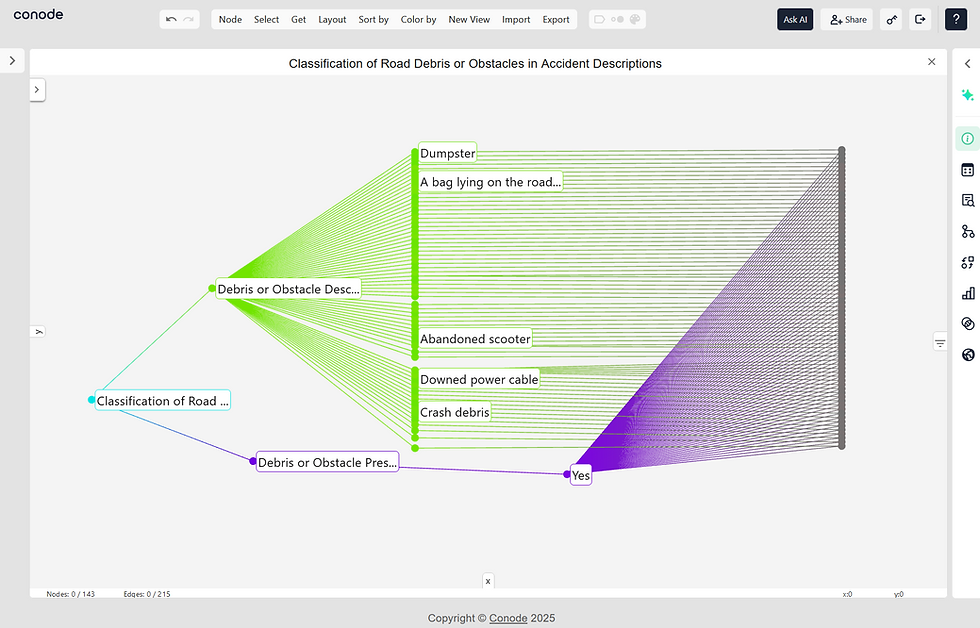

Ontology design shouldn’t be a bottleneck. With Conode, you can move from raw data to structured insights instantly—without months of manual schema engineering.
In our next blog, we’ll dive deeper into the Conode Extract Agent, which lets you dynamically create ontologies from unstructured text, no coding required. Stay tuned!
Comments